Business Analytics Roundtable
The Power of Business Analytics
Join the Business Analytics Initiative at the Poole College of Management for its annual Business Analytics Roundtable. This roundtable will feature thought leaders from academia, firms, and organizations that are working on state-of-the-art analytics and the transformational power of those analytics. The goal for the event is for experts to share emerging trends in business analytics, generate ideas, and create connections for the future.
Students, Faculty/Staff, and Corporate Partners are welcome to attend.
Date: April 17, 2024
Time: 9:15 am – 3:15 pm (includes pre-meeting breakfast at 8:30 am and networking lunch at 11:45 am)
Location: James B. Hunt Jr. Library (Duke Energy Hall)
The Business Analytics Initiative (BAI), housed in the Poole College of Management at NC State University, serves as the hub for all of our business analytics activities including:
- Providing a world class business education that enables our graduates to tackle today’s challenging data-driven business problems.
- Engaging in world-class research that develops innovative methodologies, frameworks, and tools to help organizations address their challenges head-on
- Delivering global thought leadership to industry and providing a forum to discuss their analytics challenges with one another.
Want to learn more?
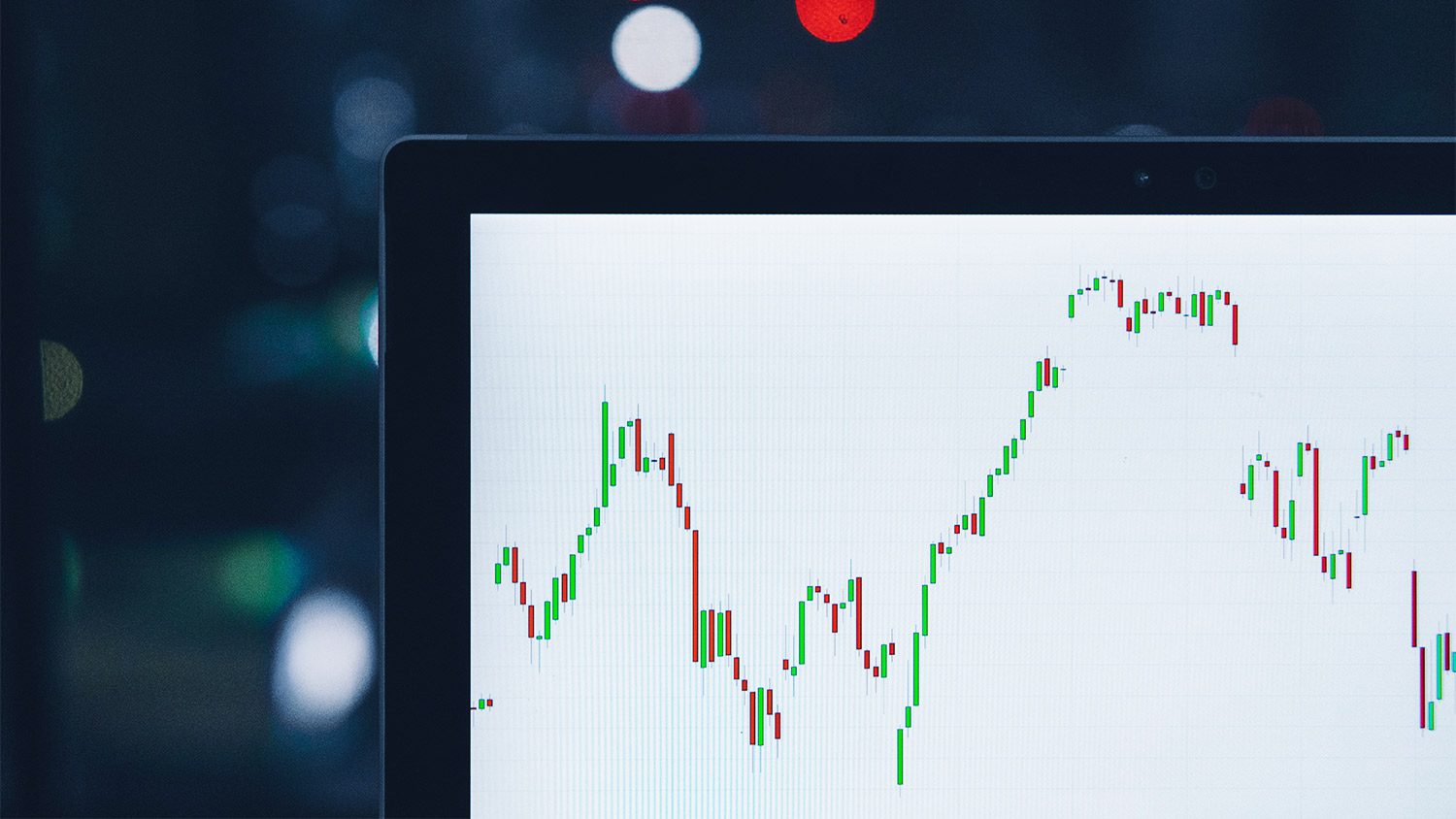
Our Mission
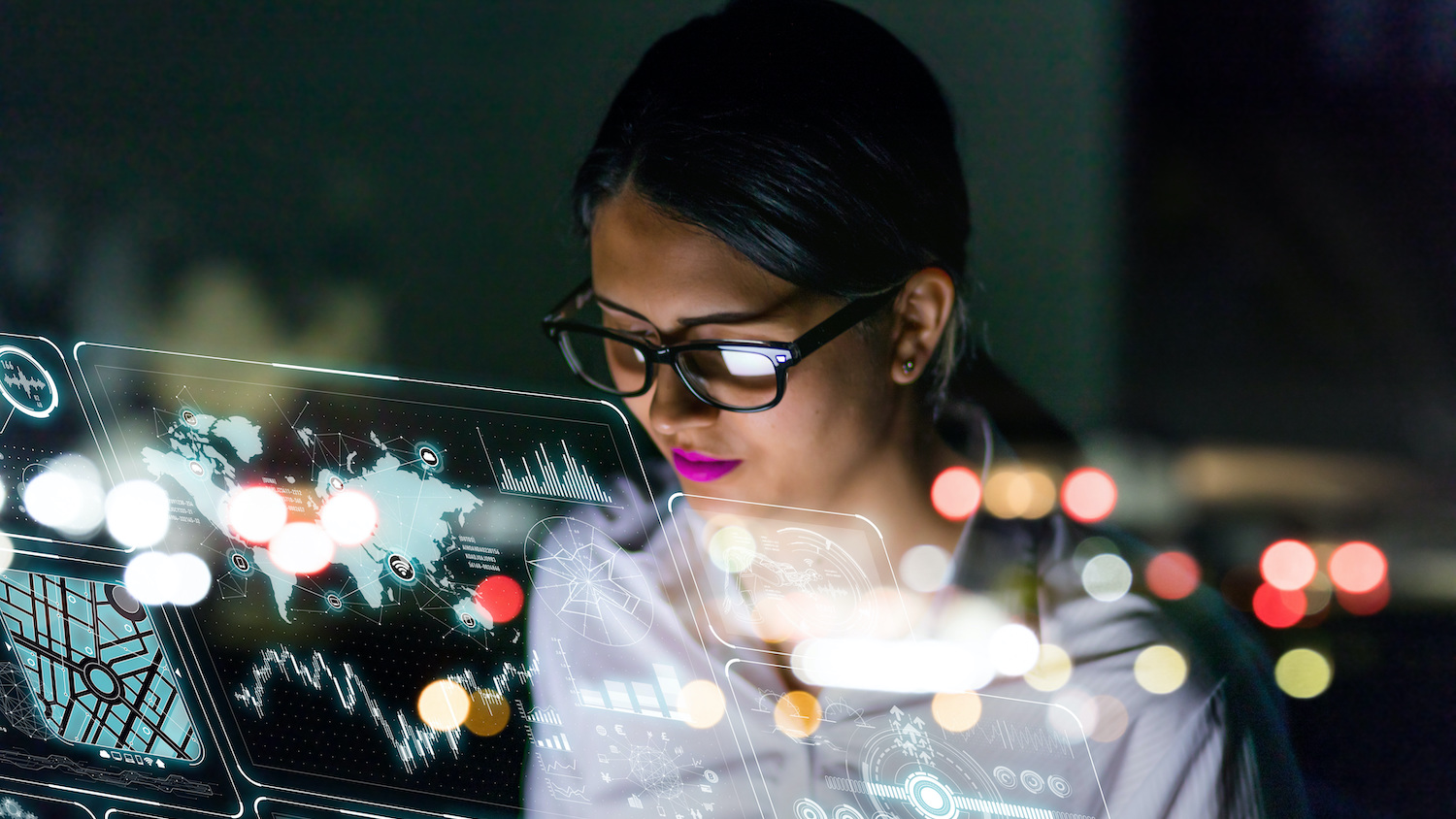
Our Vision

Our Values
Business Analytics has the potential to transform the modern organization — making the organization more efficient, robust and successful than ever before. To make this transformation, we need to take advantage of the volume, velocity and variety of modern data. The BAI will train students, conduct research and provide insights into how to transform large-scale data, in all its forms, from raw data to the wisdom necessary to guide their organizations.
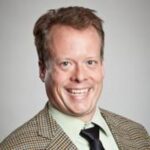
McLauchlan Distinguished Professor of Marketing and Analytics, Poole College of Management